I don’t think anything makes me crazier than when a business leader receives critical data and instead of acting and increasing returns, they go ahead and design a “test” that derails everything. Digital marketing & business analytics are powerful precisely because they generate thousands of signals that can improve outcomes at a scale far outpacing any prior method. Most businesses are sitting on data that contains all of the information required to improve business outcomes materially. The problem is, they just don’t seem to know it. Let’s explore how to know when to test, versus when to act. It’s probably the most important digital skill your business organization needs to master.
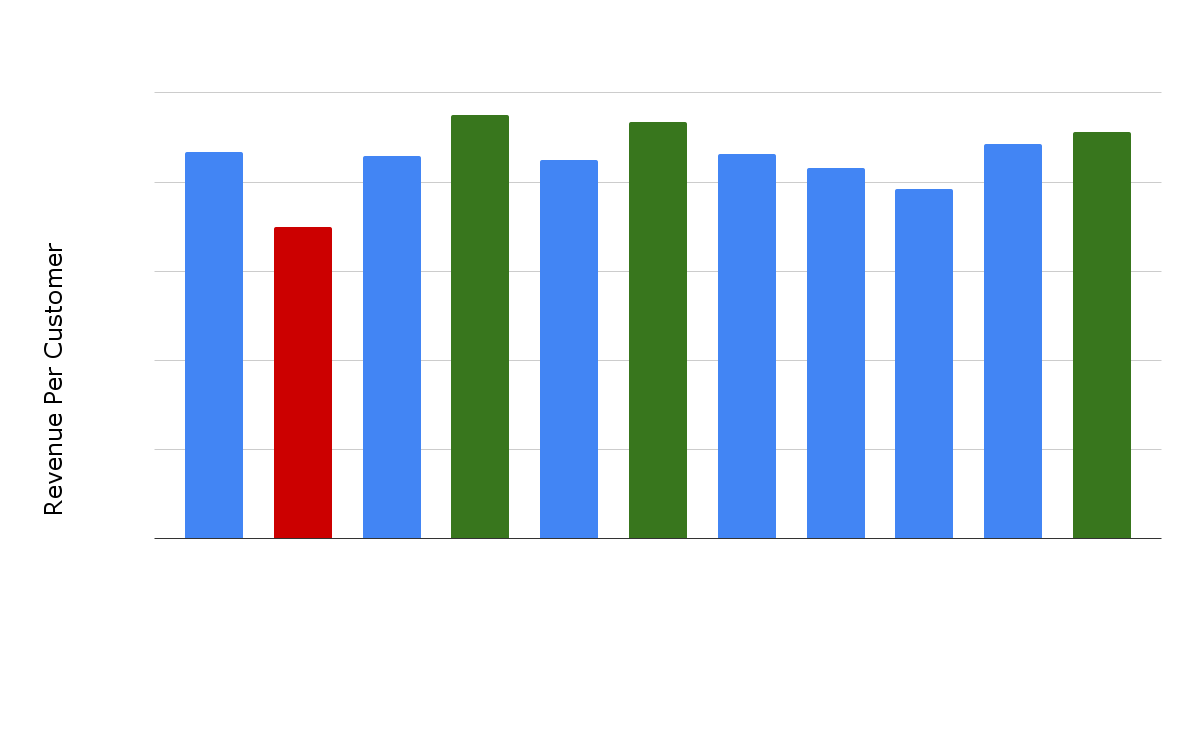
Here we have twelve customer segments, and their corresponding revenue-per-customer.
How many of you have looked at this data and recommended the following test:
“We’d like to design a test to see whether or not the segments in green are more valuable customer segments than the segment in red. To test this, we are going to run a randomized test-control multi-variate, multi-arm-bandit monte-carlo…{insert your favorite jargon to confuse your boss and get approval here}”
A customer segment with a higher revenue-per-customer than another segment is more valuable. This doesn’t require a test – it’s simply the descriptive fact.
Actionable descriptive statistics are called signals.
If your digital marketing technologies allow for investment weighting towards these more valuable customer segments, you should simply deploy those weighted changes. If your customer segments change in value over time, then you can re-calibrate that investment accordingly. This will improve overall outcomes in all cases. This is how signals turn into business returns.
Here is some other data. It is not a signal.
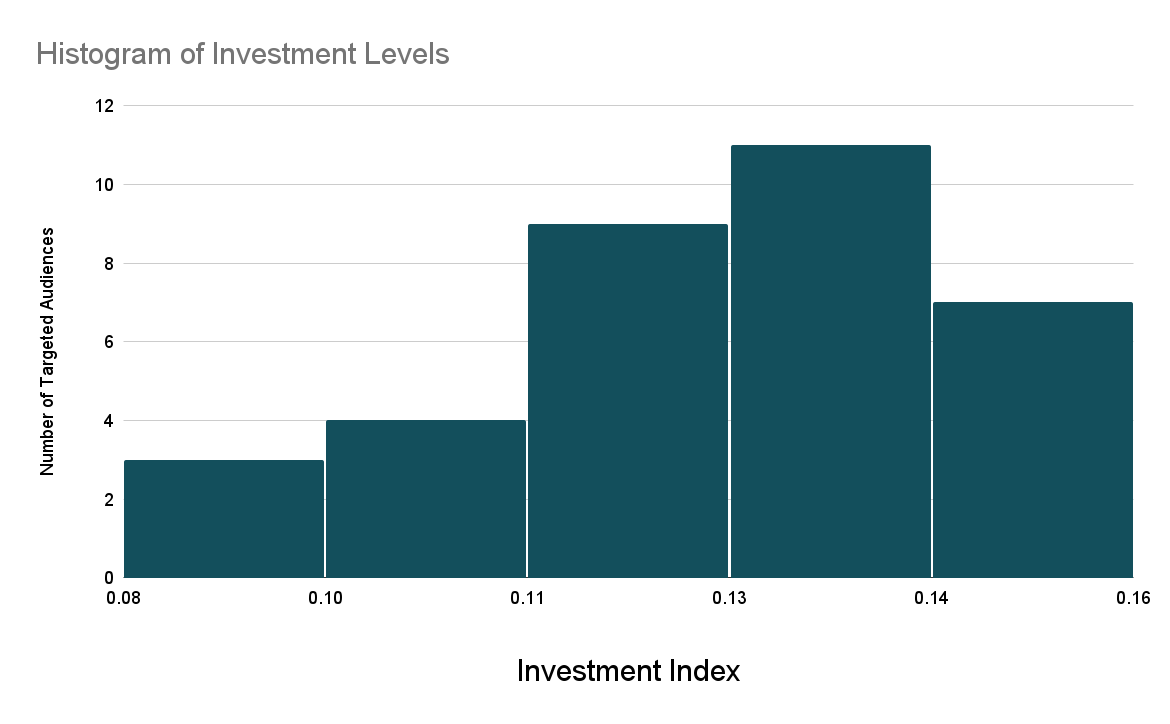
Why is there no signal in this data? It’s equivalently factual. That is not the only standard.
Are the target audiences of varying values? We do not know.
Are the audiences with the highest investment the most valuable? Again, we do not know.
Data without a known causal framework or the requisite complementary information are great fodder for a strategic test.
WHAT ARE GOOD MARKETING TESTS THAT THIS DATASET SUPPORTS DEPLOYING?
- Would the business performance improve if we increased the investment index to the highest level across all audiences?
- Would the business profitability increase if we decreased the investment index to the lowest level across all audiences?
- What are the different return levels seen in practice when investing at various levels?
This is a great use of testing because the data:
- Don’t contain any inherent strategic conclusions. What’s the right investment level by audience? No one could know that simply by looking at this dataset alone.
- Can frame a practical, measurable strategic change and give rise to a method for measuring the test outcome. – This data leads to an easy-to-design test that’s taken right from the real world: “Try investing at the 0.08 levels for half of the audiences at 0.13, and see if the total business return changes between the two groups.”
- Have a clear “If the hypothesis is rejected, do this – if the hypothesis is accepted, do that.” – If we used this information to run a test that showed: when investing across all audiences at the highest investment index, business returns doubled, it would be a slam dunk as to what to do going forward!
The idea that digital business analytics data always require rigorous testing to drive business outcomes is complete nonsense. Knowing what data are signals and which simply uncover test potential is easy in principle, clearly difficult in practice. How do you solve this quickly and reliably? Simple – find someone who understands the underlying principles behind the source of your data (say, customer sales data) and recipient of your considered action (say, programmatic media campaign/platform). If your organization doesn’t have any domain experts in both, your organization is too siloed, and we both now know your most critical hiring need.