If we can grasp that measurement and attribution are different things, data might truly transform recovery from this pandemic, and we might realize an age of abundance across industries, companies, and people – collectively improving all our lives.
Today there was an incredible example of measurement and attribution collaboration published in the New York Times – showing how US citizen’s have altered their travel behavior – or haven’t – down to the county level alongside the context of the state-by-state variation on government response, particularly “stay at home” directives.
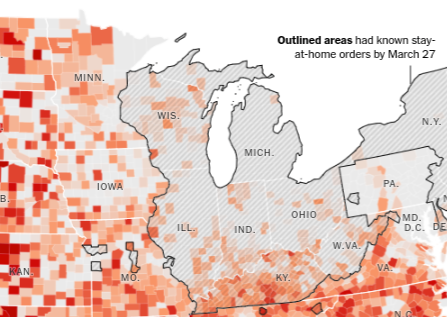
a snapshot from the incredible data-viz: Full view available in link to article (Image: The New York Times)
The attribution experts – lead by the authors of the piece – James Glanz, Benedict Carey, Josh Holder, Derek Watkins, Jennifer Valentino-DeVries, Rick Rojas and Lauren Leatherby collaborated with the measurement experts – in this case it was technology firm Cubeiq, whose data articulated how behavior has changed in the US in March.
These combined efforts and expertise allowed for exceptionally specific and useful predictions about where COVID-19 cases are likely to continue to accelerate at rates unabated, where case growth is likely to decelerate first, and what signals we need to see in “user behavior”, in this case, everyone in the US, to slow the pandemic, flatten the curve, and retain as much health and vitality of our population as possible.
It is great that the Times published this piece. It’s likely to stimulate behavior that will improve outcomes. It’s also tragic that this piece came on April 2nd, 2020. The US already has well over 200,000+ positive COVID-19 cases, and will see many more before this crisis crests.
Why haven’t we seen analysis and insights like this sooner? Why are government responses full of generalities (“the situation is evolving”) , vague references (“we don’t really know how many people have symptoms”), and why are so many experts (Dr. Fauci & many others) who are perfectly capable of giving more precise advice relegated to saying “we need your help”?
It is not a failing of our experts and the greatest minds in the public health domain, this is a failing of our collective approach to measurement and attribution. Untold troves of incredible measurement and information are locked away within measurement tools, firms, and technologists who’ve enabled incredibly robust data collection, but have no expertise in how best to utilize or share said measurements.
In this particular crisis, epidemiologists and their teams have no standard access or insight into the measurement data potentially available at their fingertips across sources public or private: what the data is, how it’s aggregated, who’s collected it and how to get access. Without any standard for data collection transparency and accuracy in today’s digital information age, there’s no signposts, no roadmap telling our measurement and domain experts how to quickly and effectively organize and collaborate to drive success. That’s why even in 2020, it takes months after a global pandemic has started to see such great work come to the front of an established voice like the New York Times: it takes incredible effort and ingenuity from the authors of this piece to navigate this without the tools we’d have if measurement and attribution were understood as separate but needed disciplines of critical collaboration.
“ELIMINATING DATA IS NOT THE ANSWER”
The environment today – data collection without consent and transparency – has limited our collective trust in technology, big data and the platforms that own and collect much of the digital datapoints about our lives. This reticence is well earned, for two key reasons. First, we are all inherently wary of the unknown. No one particularly afraid of the silly ad for shoes that follows you around the internet, but since you don’t know how that ad is finding you and who is responsible for serving it, you are left to wonder, “What do they know about me? What data of mine is out there? Who has it?” None of those questions generate feelings of comfort and security.
Second, since data & measurement technologies aren’t transparent about their collections, methods, and results, they are often granted the task of coming up with the decisions and outcomes from the data they’ve collected. An ad for something you don’t want following you around the internet is a waste of money, and a bad experience for the user but also for the brand that pays for the exposure. Why are such dumb things being done with our data? This is because without data & measurement transparency, we’ve ceded the responsibility of answering “why” and “what should we do” – the role we’d typically ascribe to a coach, teacher or strategist – to the people who collect all of the data.
And while in sports we seem to intuitively understand that coaching is not simply keeping score, we don’t seem to grasp this concept more broadly. The ability to answer the question “what happened” does not make one an expert in using that data to describe what should or could be done. But poor decisions coerced from ill-equipped measurement specialists are not an argument against measurement. Reactions such as “do not track”, the “right to be forgotten”, ad blockers and a myriad of other responses to this situation should certainly be our rights as free citizens, but must also not be mistaken as the answer to improving our lives and solving the problems that the digital information age has yet to solve. We shouldn’t stop collecting information. We shouldn’t stop sharing information. Society was not better off before we had all of this data. This reaction speaks to our instincts and our emotions, but no great coach would ever ask their staff to stop keeping score. We need to lean into measurement as much as ever. But what we need to stop placing the responsibility of answering “why did this happen?” and “what should we do?” on measurement companies, technologies, and practitioners.
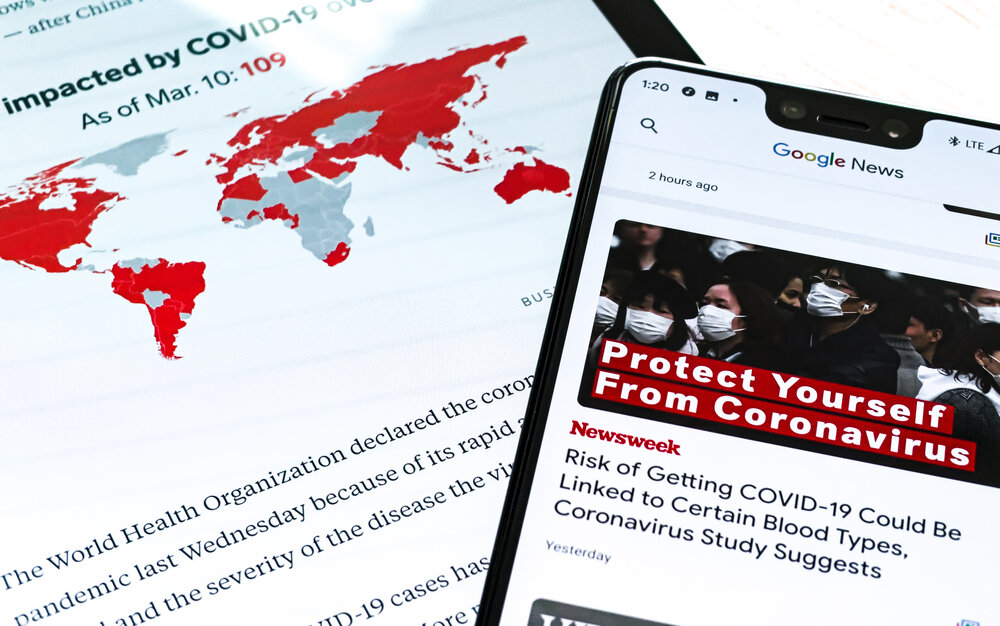
How many epidemiologists globally could design the world’s best contagion models, forecasts and predictions if only they had better data?
Did they know in Italy, many months ago, of specific travel data from a company like Cubeiq? I’m sure connections of similar ilk were made with research teams in certain cases, but I would bet most-to-all of these opportunities to connect the best data with the best talent were missed. And that’s not an Italy or Europe problem, it’s an everywhere problem. Our collective zeitgeist hasn’t yet committed to a mandate of transparent data collection and articulation. We have not yet admitted that companies collecting data do not know the best methods for utilizing that data to improve outcomes for themselves or others.
Pervasive and systematic data collection can drive exceptional outcomes for users, contractors, businesses, and societies – but only if there’s transparency about the data collected. What data is known? What don’t we know? Data collectors and technologies must not only be transparent about the data collection, but also deliberate about articulating designs and definitions. “Data dictionaries” shouldn’t be only the domain of IT professionals, data scientists, analysts or technologists. Every business collecting data needs to understand their “data dictionary”, take the time to articulate that model to their users and partners, and be specific about how it’s collected, stored and aggregated. And this isn’t about a public mandate for full-scale data sharing from any company to another at any time – far from it. Data should remain private and consent should always be involved. It’s the methods, schema and concepts of what you are collecting and storing that should be more transparent and discoverable. Outcomes don’t improve, in business, health, or otherwise, when data we needed isn’t discovered until it’s months too late.
“DOMAIN EXPERTS KNOW THE ANSWER TO “WHY?””
The best resource for answering your attribution questions, “why did this happen?” and “what should we do next?” vary in each and every example, but the thread that ties them is this: domain expertise. Currently, organizations that understand this lap their respective fields. These outliers already understand that measurement and attribution are very different things, they need both to succeed, but that measurement tools can’t solve “why” questions, and difficult “why” questions can’t be solved without better measurement.
Ever wonder why executives from these companies – whether it be titans like Expedia or Amazon, or niche organizations like Doctors Without Borders or the Boston Red Sox, always answer the question “Does your organization look to analytics or prior expertise?” with “Our approach is a blend of both”? What’s not well articulated in this soundbite is that it isn’t just a random “mix” – throw some data in here, throw some grizzled experts there – it’s a specific recipe that gets the job done. Measurement is deliberate, transparent, and organized in a way that’s visible and useful for the company’s domain experts. Domain experts then apply their years of understanding and knowledge to articulate specific, nuanced strategies about what to do next. I’m no expert in the vast majority of my examples, but in one example I can imagine a conversation going something like this:
“We’re struggling against our division opponents Amy, what can you show us about their pitching?”
— Mikey Manager, Red Sox
“I’ve tabulated the pitch F/X data by pitch type and game situation for our division opponents, putting their location and velocity numbers versus the league averages. The first page has data on the 10 pitchers we will probably face next.”
— Amy Analytics President, Red Sox
“Seeing this, it’s clear that they are pitching us up and out of the zone with hard stuff more than the rest of the league. We need to layoff those pitches. Holly, do you agree?”
— Mikey Manager, Red Sox
“True Mike, but it’s not that simple. Roberts and Smith are two of the best high fastball hitters in the league. I can’t tell them to layoff that pitch. But I do think they’re chasing those more because they’ve been struggling with offspeed away. Let me share this with them and I’ll start zone discipline drills focused on curveballs and sliders with the hitters.”
— Holly Hitting Instructor, Red Sox
None of the domain experts could solve what they were seeing – poor hitting versus their division rivals. It took exceptional measurement expertise – Amy Analytics President in this case, to diagnose the measurement needs and deploy the right report to the group. But the actions weren’t a simple recitation of “Stop doing that!” to the observed problem. It took deep domain expertise – in this case two coaches with domain experience, to think through the potential treatments, their implications, and the best path to solving the problem.
“Measurement != Attribution”
Let’s bring this back to digital business attribution and analytics. It’s currently still the industry standard to call measurement – or aggregated data, reported in a structure – as attribution. At Bonsai, if we ask 100 companies to share an example of their marketing attribution, 99 of them will send us a report of measurement data. Few, if any, will include any evidence & domain-based insight into why their unique business outcomes occurred, and what they can do about it to generate more of them. How do we know? These reports will invariably look the same: tables of data, organized and curated, with outcomes and inputs aligned by data design rules, often dictated by the easiest path to measure. Rarely, maybe 1 of a 100, will include domain expertise or evidence – clearly coming from those who have insights into “why” – that dictates particular aspects of their model that makes it uniquely productive. There’s a very good reason that at Bonsai, we have a separate services for measurement and attribution: they are simply different things.
“Measurement Expertise Is Executive Level”
Measurement platforms and stakeholders need to come clean: YES, technology companies are collecting an incredible amount of data, but NO, they do not know what to do with it. As a community we also need to come to terms with this, without pitchforks and torches, and productively move forward with better transparency and cross-domain partnerships. Our domain experts require the best measurement money can buy. Our measurement experts require the space, respect and freedom to do that job without fear of reprisal. And measurement experts sharing data dictionaries and records with domain experts isn’t a secession of value or importance. There’s a reason the coach isn’t keeping score – she’s not very good at it. A coach with an exceptionally proficient measurement staff is going to run circles around a team without one. As technology allows us to collect more data, measurement expertise should rise to the equivalent as every other domain expertise within organizations. “Analytics managers” shouldn’t stop at “Vice President”, and they shouldn’t be reporting to domain experts. Measurement expertise is President-level value, reporting only to the top, in every industry – full stop.
How can you know if measurement expertise is valued appropriately at your company? One way you can tell is by looking at the entry-level job titles: do people at your company start as “Analysts”? Then you know your organization both doesn’t value measurement expertise enough. For many industries, this is more than a problem at one company, this is a problem across the entire industry. And in places where measurement expertise isn’t valued highly enough, you can bet that they are asking these same people to make decisions they aren’t equipped to answer. They are being set up to fail.
So take this time you have to reflect about your company. How are you thinking about measurement and attribution? Do your measurement teams have the adequate gravitas they need to hold the biggest seats at the table? Do your domain experts have immediate access to the datasets they need to make the right judgments? Does your process ask decisions of measurement experts that they cannot fairly be asked to answer? Now is the time to examine your processes – and make the situation better for the next time around.